[Other] MM1: Methods, Analysis & Insights from Multimodal LLM Pre-training
Abstract: In this work, we discuss building performant Multimodal Large Language Models (MLLMs). In particular, we study the importance of various architecture components and data choices. Through careful and comprehensive ablations of the image encoder, the vision language connector, and various pre-training data choices, we identified several crucial design lessons. For example, we demonstrate that for large-scale multimodal pre-training using a careful mix of image-caption, interleaved image-text, and text-only data is crucial for achieving state-of-the-art (SOTA) few-shot results across multiple benchmarks, compared to other published pre-training results. Further, we show that the image encoder together with image resolution and the image token count has substantial impact, while the vision-language connector design is of comparatively negligible importance. By scaling up the presented recipe, we build MM1, a family of multimodal models up to 30B parameters, consisting of both dense models and mixture-of-experts (MoE) variants, that are SOTA in pre-training metrics and achieve competitive performance after supervised fine-tuning on a range of established multimodal benchmarks. Thanks to large-scale pre-training, MM1 enjoys appealing properties such as enhanced in-context learning, and multi-image reasoning, enabling few-shot chain-of-thought prompting.
Lay summary (by Claude 3 Sonnet): This research focuses on building high-performance multimodal large language models (MLLMs) that can understand and generate both text and images. The researchers conducted extensive experiments to understand the importance of different components of the model architecture and the types of data used for training. They found that using a careful combination of different data sources was crucial for achieving state-of-the-art performance. Specifically, they used a mix of image-caption data (images with descriptions), interleaved image-text data (images and text together), and text-only data during the pre-training stage. The image encoder (the part of the model that processes images), along with the image resolution and the number of image tokens, had a substantial impact on performance. However, the specific design of the component that connects the vision and language parts of the model was relatively unimportant. By scaling up their approach, the researchers built a family of multimodal models called MM1, with up to 30 billion parameters. These models achieved state-of-the-art performance on pre-training metrics and competitive results on various multimodal benchmarks after fine-tuning. Thanks to the large-scale pre-training, MM1 models exhibit desirable properties such as enhanced in-context learning (ability to understand and follow prompts), multi-image reasoning, and few-shot chain-of-thought prompting (ability to break down and solve complex problems with minimal examples).
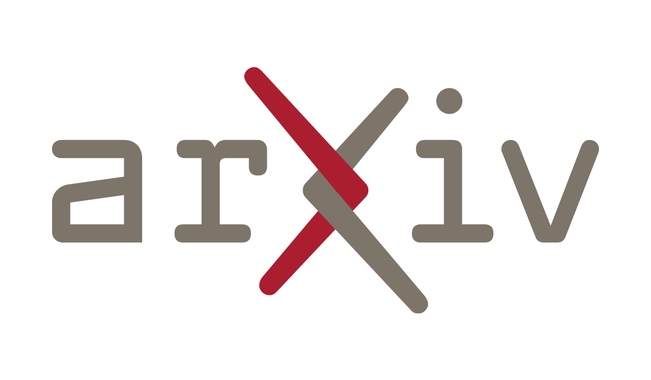
Add comment